Exploring the Use of Unsupervised Deep Learning for Robots Mimicking Human Movements
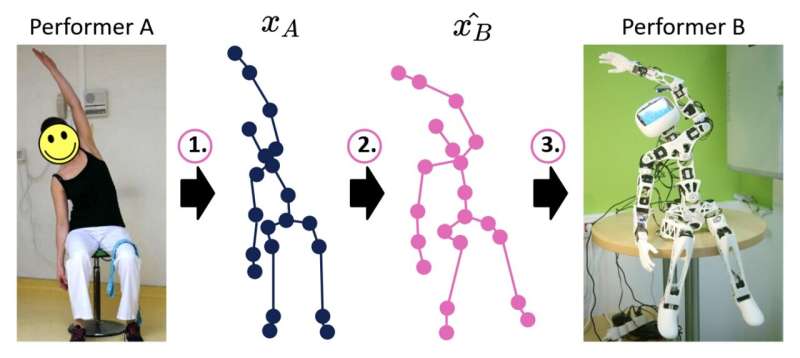
10th March 2024 feature
The following article has gone through a thorough review based on protocols and methods designed by Science X's editorial team. Points of credibility validated by the editors include:
- fact-checked
- preprint
- trusted source
- proofread
Authored by Ingrid Fadelli, Tech Xplore
Human-imitating robots could revolutionize our lives if they can learn to execute daily tasks accurately without extensive pre-programming. Despite significant progress in imitation learning technology, there remains the obstacle of inconsistency between a robot's physical characteristics and those of a human.
Deep learning-based model developed by U2IS, ENSTA Paris researchers strives to enhance motion imitation capabilities of humanoid robots. This model, currently in a pre-published phase on arXiv, addresses the issue by treating motion imitation as a three-step process, thus mitigating the human-robot correspondence problem.
'Our early-stage research works towards advancing online human-robot imitation by translating sequences of joint positions from human motions to those achievable by a particular robot, considering its physical constraints,' explained Louis Annabi, Ziqi Ma, and Sao Mai Nguyen in their paper. 'We propose an encoder-decoder neural network model performing domain-to-domain translation that leverages the generalization capabilities of deep learning methods.'
Annabi, Ma, and Nguyen's model breaks down the human-robot imitation process into three main stages: pose estimation, motion retargeting and robot control. It starts by using pose estimation algorithms to predict sequences of skeleton-joint positions as exhibited by humans.
Then, the model translates the forecasted skeleton-joint positions sequences into similar joint positions achievable by the robot. These sequences are then used to guide the robot's motions, potentially allowing it to perform the desired task.
'Training such a model would ideally involve pairs of associate robot and human motions. However, such data is hard to find, and collecting it is a tedious process. Therefore, we have turned towards unpaired domain-to-domain translation using deep learning methods for human-robot imitation,' the researchers mentioned.
In their preliminary tests, Annabi, Ma, and Nguyen assessed their model against a straightforward method that doesn't rely on deep learning to replicate joint orientations. The results did not meet their expectations, suggesting that existing deep learning techniques may be inadequate in re-targeting motions in real-time.
The team plans to perform more testing to detect any limitations before making necessary adjustments to the model. The finding so far indicates that despite unsupervised deep learning techniques can be applied to enable imitation learning in robots, they are yet to reach a performing level suitable for release on actual robots.
'Our future work is threefold: to delve deeper into the failure of the current method, to build a database of paired motion data obtained either from human-human or robot-human imitation, and to enhance the model structure to deliver accurate retargeting predictions,' the researchers concluded.
For more details: Refer to the work of Louis Annabi and team titled 'Unsupervised Motion Retargeting for Human-Robot Imitation
© 2024 Science X Network