Real-Time Sketching by Humanoid Robot: A Lifelike Approach
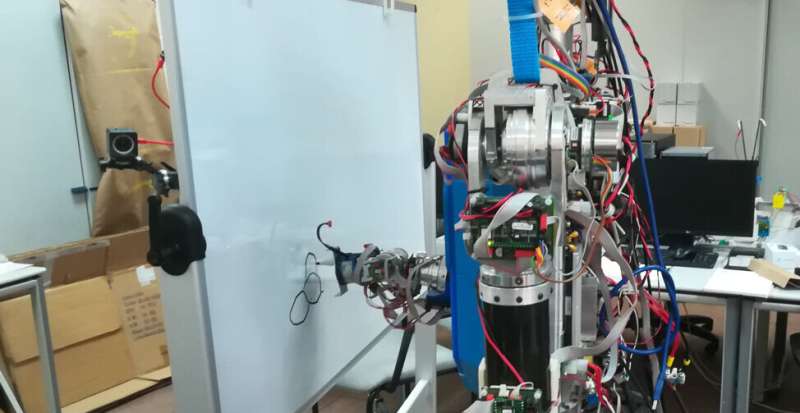
February 24, 2024 feature
This article has been reviewed according to Science X's editorial process and policies. Editors have highlighted the following attributes while ensuring the content's credibility:
- fact-checked
- preprint
- trusted source
- proofread
by Ingrid Fadelli , Tech Xplore
The rapid advancement of deep learning algorithms and generative models has enabled the automated production of increasingly striking AI-generated artistic content. Most of this AI-generated art, however, is created by algorithms and computational models, rather than by physical robots.
Researchers at Universidad Complutense de Madrid (UCM) and Universidad Carlos III de Madrid (UC3M) recently developed a deep learning-based model that allows a humanoid robot to sketch pictures, similarly to how a human artist would. Their paper, published in Cognitive Systems Research, offers a remarkable demonstration of how robots could actively engage in creative processes.
'Our idea was to propose a robot application that could attract the scientific community and the general public,' Raúl Fernandez-Fernandez, co-author of the paper, told Tech Xplore. 'We thought about a task that could be shocking to see a robot performing, and that was how the concept of doing art with a humanoid robot came to us.'
Most existing robotic systems designed to produce sketches or paintings essentially work like printers, reproducing images that were previously generated by an algorithm. Fernandez-Fernandez and his colleagues, on the other hand, wished to create a robot that leverages deep reinforcement learning techniques to create sketches stroke by stroke, similar to how humans would draw them.
'The goal of our study was not to make a painting robot application that could generate complex paintings, but rather to create a robust physical robot painter,' Fernandez-Fernandez said. 'We wanted to improve on the robot control stage of painting robot applications.'
In the past few years, Fernandez-Fernandez and his colleagues have been trying to devise advanced and efficient algorithms to plan the actions of creative robots. Their new paper builds on these recent research efforts, combining approaches that they found to be particularly promising.
'This work was inspired from two key previous works,' Fernandez-Fernandez said. 'The first of these is one of our previous research efforts, where we explored the potential of the Quick Draw! Dataset works for training robotic painters. The second work introduced Deep-Q-Learning as a way to perform complex trajectories that could include complex features like emotions.'
The new robotic sketching system presented by the researchers is based on a Deep-Q-Learning framework first introduced in a previous paper by Zhou and colleagues posted to arXiv. Fernandez-Fernandez and his colleagues improved this framework to carefully plan the actions of robots, allowing them to complete complex manual tasks in a wide range of environments.
'The neural network is divided in three parts that can be seen as three different networks interconnected,' Fernandez-Fernandez explained. 'The global network extracts the high-level features of the full canvas. The local network extracts low level features around the painting position. The output network takes as input the features extracted by the convolutional layers (from the global and local networks) to generate the next painting positions.'
Fernandez-Fernandez and his collaborators also informed their model via two additional channels that provide distance-related and painting tool information (i.e., the position of the tool with respect the canvas). Collectively, all these features guided the training of their network, enhancing its sketching skills. To further improve their system's human-like painting skills, the researchers also introduced a pre-training step based on a so-called random stroke generator.
'We use double Q-learning to avoid the overestimation problem and a custom reward function for its training,' Fernandez-Fernandez said. 'In addition to this, we introduced an additional sketch classification network to extract the high-level features of the sketch and use its output as the reward in the last steps of a painting epoch. This network provides some flexibility to the painting since the reward generated by it does not depend on the reference canvas but the category.'
As they were trying to automate sketching using a physical robot, the researchers had to also devise a strategy to translate the distances and positions observed in AI-generated images into a canvas in the real world. To achieve this, they generated a discretized virtual space within the physical canvas, in which the robot could move and directly translate the painting positions provided by the model.
'I think the most relevant achievement of this work is the introduction of advanced control algorithms within a real robot painting application,' Fernandez-Fernandez said. 'With this work, we have demonstrated that the control step of painting robot applications can be improved with the introduction of these algorithms. We believe that DQN frameworks have the capability and level of abstraction to achieve original and high-level applications out of the scope of classical problems.'
The recent work by this team of researchers is a fascinating example of how robots could create art in the real world, via actions that more closely resemble those of human artists. Fernandez-Fernandez and his colleagues hope that the deep learning-based model they developed will inspire further studies, potentially contributing to the introduction of control policies that allow robots to tackle increasingly complex tasks.
'In this line of work, we have developed a framework using Deep Q-Learning to extract the emotions of a human demonstrator and transfer it to a robot,' Fernandez-Fernandez added. 'In this recent paper, we take advantage of the feature extraction capabilities of DQN networks to treat emotions as a feature that can be optimized and defined within the reward of a standard robot task and results are quite impressive.
'In future works, we aim to introduce similar ideas that enhance robot control applications beyond classical robot control problems.'
© 2024 Science X Network