Video-based deep learning model for tracking embryonic development
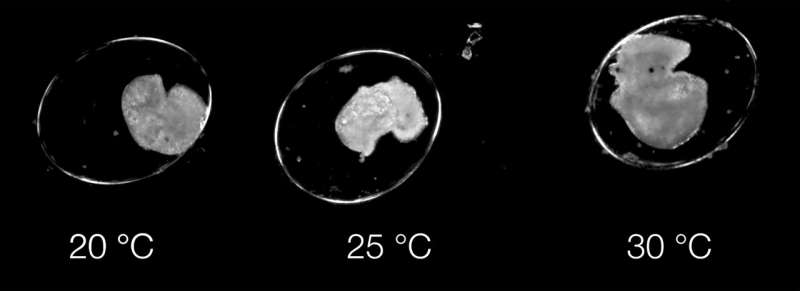
May 28, 2024
This piece of writing has undergone a thorough review based on the practices and policies crafted by Science X's editorial committee. The review ensured the validity of the article's content, focusing especially on the features mentioned below:
- fact-checked
- undergone review by peers
- information from a reputable source
- well-edited
vetted by University of Plymouth
A research conducted under the leadership of University of Plymouth revealed that a cutting-edge deep learning AI model, built specifically for video inputs, can identify sequences and timeline of events during embryonic development.
The study, entitled 'Dev-ResNet: Automated developmental event detection using deep learning,' was featured in the Journal of Experimental Biology. It demonstrates how Dev-ResNet can understand and identify key developmental events in pond snails, such as functioning of the heart, movement initiation, hatching, and even death.
A brilliant feature debuted in this study is a 3D model which analyses the transitional changes between frames of the video. This unique method enables the AI model to learn from these characteristics, as opposed to the conventional use of still imagery.
Video use allows the AI model, Dev-ResNet, to promptly detect multiple events from the early heartbeats or the emergence of movements, up to shell formation or hatching, revealing temperature sensitivities previously unknown to us.
The authors suggest that although the model was used for studying pond snail embryos in this research, its application can be more extensive, potentially across all species. They have made available detailed scripts and guidelines for applying Dev-ResNet to different biological systems.
In the foreseeable future, this method could contribute to quicker understanding of how climatic changes, among other external factors, influence humans and other animals.
The research was led by Ziad Ibbini, a Ph.D. candidate who has also studied BSc Conservation Biology at the university and taken a year off to enhance his skills in software development, before embarking on his Ph.D. venture. He was at the helm of the design, development and execution of Dev-ResNet.
Ibbini noted that outlining developmental events—deducing what occurs when in animal development—although quite challenging, holds high significance as it allows for better understanding of changes in event timing across species and environments.
He added that Dev-ResNet is a compact and effective 3D convolutional neural network, capable of understanding developmental events using videos and can be trained fairly easily on any consumer hardware.
The real challenge, according to Ibbini, lies in creation of training data for the deep learning model. He emphasizes that the model's proficiency has been proven—it simply needs the right training data.
Ibbini's objective is to equip the broad scientific community with powerful tools enabling them to better comprehend how various factors affect species' development and consequently devise strategies to safeguard them. He believes that Dev-ResNet is a massive leap in that direction.
Dr. Oli Tills, the senior author of the paper and a UKRI Future Leaders Research Fellow, stated that this research is not only crucial in technological context, but also vital for advancing our understanding of organismal development, a field in which the University of Plymouth has over two decades' experience.
Tills concluded by expressing his excitement regarding the future applications of this novel deep learning-powered capability in studying the dynamic stages in animal lives.
Journal Details: Journal of Experimental Biology
Contributor: University of Plymouth