Exploring the Scaling of Deep Learning Models for Chemistry Research
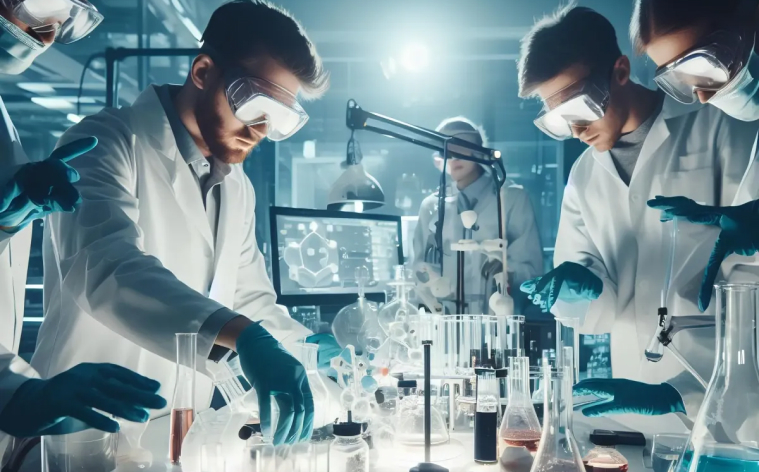
November 11, 2023
Feature
This article received a thorough review consistent with the editorial process and policies of Science X. During review, the editors ensured the credibility of the content while highlighting the following attributes:
- fact-checked
- peer-reviewed publication
- trusted source
- proofread
Article written by Ingrid Fadelli, Tech Xplore
Over the past years, deep neural networks (DNNs) have demonstrated their significant capabilities through the analysis of a massive amount of data, which could accelerate research in numerous scientific disciplines. In certain instances, computer scientists have trained models based on these networks for analyzing chemical data to identify valuable chemicals for different applications.
Researchers from the Massachusetts Institute of Technology (MIT) have recently conducted a study examining the neural scaling behavior of large DNN-based models. These models have been trained to produce favorable chemical compositions and understand interatomic potentials. Published in Nature Machine Intelligence, their paper reveals the rapid improvement in the performance of these models with an increase in their size and the dataset on which they are trained.
For their research, the team drew inspiration from 'Scaling Laws for Neural Language Models' by Kaplan et al., which established that increasing the size of a neural network and the volume of data used in its training leads to predictable advancement in the model. The motive of their research was to check whether neural scaling could be applied to models trained on chemistry data for applications such as drug discovery, explained Nathan Frey, one of the researchers.
The commencement of the research project dates back to 2021. Therefore, it predates the release of highly prominent AI-based platforms like ChatGPT and Dall-E 2. During that period, the prospective upscaling of DNNs was considered crucial to a handful of fields. However, few investigations had been carried out exploring their scaling in the physical or life sciences.
The study undertaken by the researchers examined the neural scaling of two unique models for the analysis of chemical data. These include a largely diversified language model (LLM) and a model based on a graph neural network (GNN). The two different types of models can be utilized to determine chemical compositions and comprehend the potentials among various atoms in chemical substances.
The team studied two distinct models: 'ChemGPT', an autoregressive, GPT-style language model built by them, and a GNN family. ChemGPT was trained similarly to ChatGPT but attempts to predict the next token in a string representing a molecule. GNNs are trained to predict a molecule's energy and forces, Frey elucidated.
The team explored the scalability of the ChemGPT model and GNNs and found that increasing the size of the model or the dataset used to train it had a significant effect on several essential metrics, revealing a rate at which the models improve as they grow larger and are trained with additional data.
'We've identified a 'neural scaling behavior' in chemical models similar to the scaling behavior seen in LLMs and vision models developed for various uses,' Frey stated. The team also demonstrated immense potential for further scaling of chemical models. Enhancing the physics of GNNs through a property called 'equivariance' can significnatly improve scaling efficiency. This achievement is stimulating because it is challenging to discover algorithms that modify scaling behavior.
The team provided a newfound perspective on the potential of two AI model types for conducting chemical research. They showed to what extent the performance of these models can be improved through scaling. This study might inspire further studies to explore the capabilities and scope for improvement of these models along with other DNN-based techniques for specific scientific purposes.
'Since our paper was first published, there has been some intriguing follow-up work analyzing the capabilities and limitations of scaling for chemical models,' Frey further added. 'More recently, I have also been concentrating on generative models for designing proteins and considering how scaling affects models for biological data.'
© 2023 Science X Network