New transistor inspired by the human brain revolutionizes AI
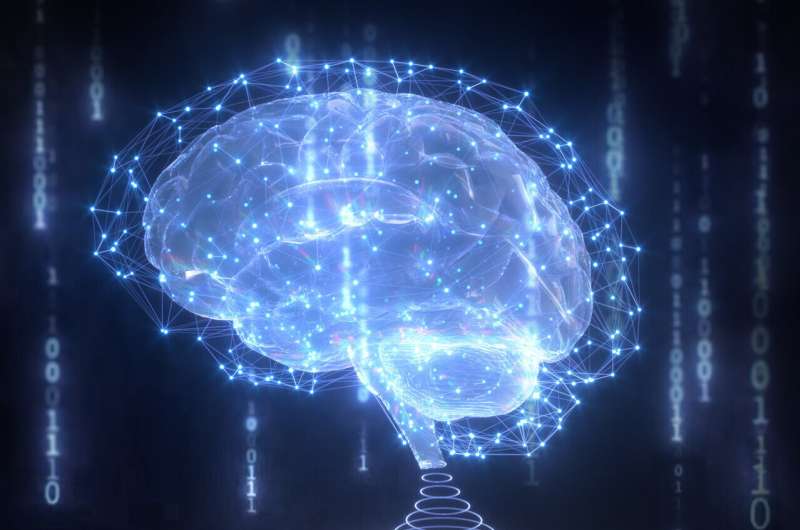
An innovative synaptic transistor that simulates the human brain's integrated processing and memory functions has been developed by researchers. This energy-efficient device can undertake complex cognitive tasks such as associative learning and operates at room temperature, marking a significant step forward in the field of artificial intelligence. Image Credit: Xiaodong Yan/Northwestern University
Inspired by the intricate functions of the human brain, a group of researchers from Northwestern University, Boston College, and the Massachusetts Institute of Technology (MIT) have engineered a ground-breaking synaptic transistor.
This cutting-edge device not only processes but also keeps data, reflecting the multifaceted role of the human brain. The recent experiments by the team demonstrated that this transistor transcends standard machine-learning chores to categorize data and can perform associative learning.
Despite the fact that past studies have used comparable tactics to construct brain-like computing gadgets, those transistors were unable to operate unless under cryogenic temperatures. However, this innovative device is stable at room temperatures, operates swiftly, uses minimal energy, and safeguards stored data even when power is cut off, making it suitable for practical applications.
The study was published in the journal Nature recently.
In the words of Mark C. Hersam, a Northwestern professor who co-led the research, the architecture of the brain is fundamentally different to a digital computer. In a digital computer, data flows between a microprocessor and memory, which consumes lots of energy and creates a bottleneck when attempting to perform multiple tasks simultaneously. However, in the brain, memory and processing of information are co-located and fully integrated, resulting in far greater energy efficiency. The synaptic transistor they developed mimics the brain by achieving concurrent memory and information processing functionality.
Hersam holds the Walter P. Murphy Professorship of Materials Science and Engineering at Northwestern’s McCormick School of Engineering. In addition to serving as chair of the department of materials science and engineering, director of the Materials Research Science, and Engineering Center, he is also a member of the International Institute for Nanotechnology. The research was co-led by Qiong Ma of Boston College and Pablo Jarillo-Herrero of MIT.
Current progress in artificial intelligence (AI) has spurred researchers to develop computers that operate similarly to the human brain. Traditional digital computing systems have distinct storage and processing units, making data-heavy tasks require a lot of energy. As smart devices constantly gather huge quantities of data, researchers are looking for novel methods to process this information without consuming too much power. The most advanced technology capable of performing combined processing and memory functions currently is the memory resistor or “memristor”. However, memristors still struggle with energy-extensive switching.
According to Hersam, despite having made significant progress by packing more transistors into integrated circuits using the same silicon architecture, this strategy still leads to high power consumption, especially in the era of big data where digital computing could overwhelm the grid. It is necessary to rethink computing hardware, especially for AI and machine-learning tasks.
To alter this paradigm, Hersam and his team explored new advancements in the physics of moiré patterns, a type of geometrical design formed when two patterns overlay each other. When two-dimensional materials are stacked, new properties not found in a single layer appear. When these layers are twisted to form a moiré pattern, electronic properties become highly tunable.
The researchers used two different types of atomically thin materials for the new device: bilayer graphene and hexagonal boron nitride. By stacking and twisting these materials, they created a moiré pattern. They could fine-tune different electronic properties in each graphene layer by rotating one layer relative to the other, even though these layers are separated only by atomic-scale dimensions. The right amount of twist enabled the creation of a neuromorphic functionality moiré physics at room temperature.
Hersam added, “Introducing twist as a new design parameter, the possibilities are extensive. Graphene and hexagonal boron nitride are structurally very similar but just different enough that you get incredibly strong moiré effects.”
To test the transistor, Hersam and his team trained it to recognize similar — but not identical — patterns. Just earlier this month, Hersam introduced a new nanoelectronic device capable of analyzing and categorizing data in an energy-efficient manner, but his new synaptic transistor takes machine learning and AI one leap further.
“If AI is meant to mimic human thought, one of the lowest-level tasks would be to classify data, which is simply sorting into bins,” Hersam said. “Our goal is to advance AI technology in the direction of higher-level thinking. Real-world conditions are often more complicated than current AI algorithms can handle, so we tested our new devices under more complicated conditions to verify their advanced capabilities.”
First, the researchers showed the device one pattern: 000 (three zeros in a row). Then, they asked the AI to identify similar patterns, such as 111 or 101. “If we trained it to detect 000 and then gave it 111 and 101, it knows 111 is more similar to 000 than 101,” Hersam explained. “000 and 111 are not exactly the same, but both are three digits in a row. Recognizing that similarity is a higher-level form of cognition known as associative learning.”
In experiments, the new synaptic transistor successfully recognized similar patterns, displaying its associative memory. Even when the researchers threw curveballs — like giving it incomplete patterns — it still successfully demonstrated associative learning.
“Current AI can be easy to confuse, which can cause major problems in certain contexts,” Hersam said. “Imagine if you are using a self-driving vehicle, and the weather conditions deteriorate. The vehicle might not be able to interpret the more complicated sensor data as well as a human driver could. But even when we gave our transistor imperfect input, it could still identify the correct response.”