Discovering new drug design opportunities with machine learning models
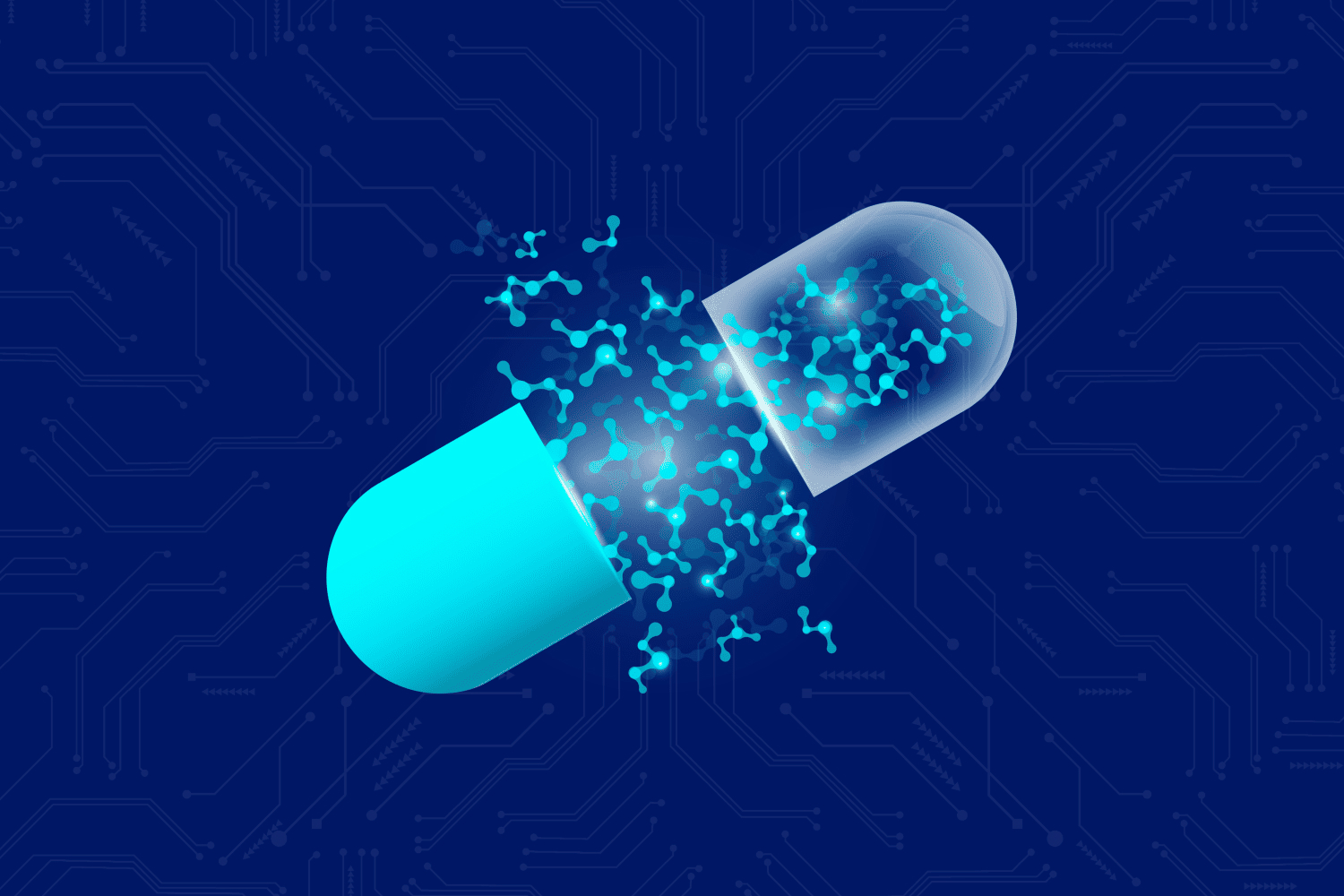
May 14, 2024
In an article that went through the editorial process of Science X and confirmed for credibility by fact checks, peer reviews, and thorough proofreading, editors highlight the use of machine learning in antibiotic development. The research was managed by the Los Alamos National Laboratory.
The adaptability of pathogens in protecting themselves from antibiotics has become quite a concern in public health. In response to this, a research team under the management of Los Alamos National Laboratory used machine learning, a form of artificial intelligence, to detect various molecular properties. The goal is to guide the research towards the development of new antibiotics, particularly targeting pathogens that are considered critical by the World Health Organization due to their high bacterial resistance.
The research was recently published in the Communications Chemistry journal.
At Los Alamos, scientist Gnana Gnanakaran explained that "some pathogens are very effective at resisting antibiotics due to their properties." Detecting specific compounds that can penetrate and inhibit some of these pathogens is difficult. The heterogeneity and depth of the chemical space are huge, and the molecular interactions across bacterial membranes are highly complex. However, the machine learning approach they use can probe the bacteria-specific, molecular-level profiles that could form the basis for successful drug development.
Gram-negative bacteria have a less permeable outer membrane, and can expel compounds that manage to infiltrate. This limits an antibiotic's effectiveness.
Data-fuelled models could help identify molecular properties that can break the bacteria's defense. However, making these calculations requires an extensive amount of computing resources, as chemically diverse compounds have a wide range of relevant properties. The machine learning-based study reduced the relevant properties spectrum and set empirical rules predicting the compound's ability to penetrate the bacteria's outer membrane.
The research team focused on Pseudomonas aeruginosa, a gram negative bacteria, and created a machine learning model to identify relevant descriptors for various compounds. The descriptors predict the success of the compounds in penetrating the outer membranes of the bacteria and avoiding expulsion. The team used high-performance computing resources at Los Alamos to analyze the molecular properties of permeation from simulations considering 1,260 chemically diverse compounds' journey through the bacterial membrane.
Through this study, important insight has been provided about the key properties that drug candidates require to penetrate Pseudomonas aeruginosa effectively. This study paves the path for similar data-driven studies in other gram-negative pathogens.
Gnanakaran highlighted the promising nature of the machine learning techniques used in the study. He believes they could be applied to similar data-driven studies in other biological membranes, including the blood-brain barrier.
Additional details are available in "Predicting permeation of compounds across the outer membrane of P. aeruginosa using molecular descriptors" in the Communications Chemistry journal, 2024.
This study was facilitated by the Los Alamos National Laboratory.