Improving Robotic Object Grasping through Deep Learning
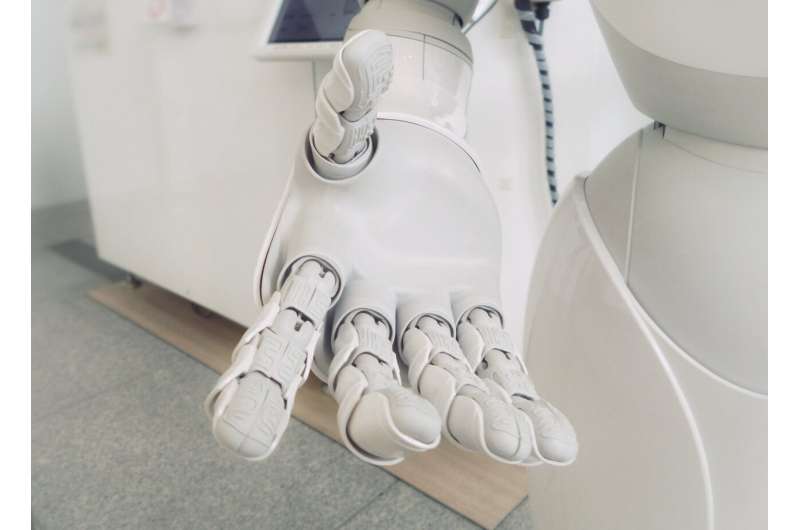
August 22, 2023
feature
This article has been reviewed according to Science X's editorial process and policies. Editors have highlighted the following attributes while ensuring the content's credibility:
- fact-checked
- preprint
- trusted source
- proofread
by Ingrid Fadelli, Tech Xplore
Most adult humans are innately able to pick up objects in their environment and hold them in ways that facilitate their use. For instance, when picking up a cooking utensil, they would normally grab it from the side that will not be placed inside the cooking pot or pan.
Robots, on the other hand, need to be trained on how to best pick up and hold objects while completing different tasks. This is often a tricky process, given that the robot might also come across objects that it never encountered before.
The University of Bonn's Autonomous Intelligent Systems (AIS) research group recently developed a new learning pipeline to improve a robotic arm's ability to manipulate objects in ways that better support their practical use. Their approach, introduced in a paper published on the pre-print server arXiv, could contribute to the development of robotic assistants that can tackle manual tasks more effectively.
'An object is grasped functionally if it can be used, for example: an index finger on the trigger of a drill,' Dmytro Pavlichenko, one of the researchers who carried out the study, told Tech Xplore. 'Such a specific grasp may not be always reachable, making manipulation necessary. In this paper, we address dexterous pre-grasp manipulation with an anthropomorphic hand.'
The recent paper by Pavlichenko and co-author Sven Behnke builds on the AIS group's previous research efforts, in particular a paper presented at the 2019 IEEE-RAS International Conference on Humanoid Robots in Toronto. As part of this past study, the team developed a sophisticated approach for the dual-arm robotic re-grasping of objects that relied on multiple complex hand-designed components.
'The motivation for our new paper was to replace such a complex pipeline with a neural network,' Pavlichenko explained. 'This reduces complexity and removes hardcoded manipulation strategies, increasing the flexibility of the approach.'
The simplified pre-grasp manipulation approach that the researchers introduced in their new paper relies on deep reinforcement learning, a highly performing and well-known technique to train AI algorithms. Using this technique, the team trained a model to dexterously manipulate objects before grasping them, ensuring that the robot is ultimately holding them in effective ways, exactly as requested.
'Our model learns utilizing a multi-component dense reward function, which incentivizes bringing an object closer to the given target functional grasp by finger-object interaction,' Pavlichenko said. 'Combined with a GPU-based simulation Isaac Gym, learning can be done quickly.'
So far, the researchers evaluated their approach in a simulation environment known as Isaac Gym and found that it achieved highly promising results. In their initial tests, their model allowed simulated robots to learn how to move distinctly shaped objects in their hands, eventually figuring out the best way to manipulate them without requiring human demonstrations.
Notably, the learning approach proposed by Pavlichenko and his Behnke could easily be applied to a variety of robotic arms and hands, while also supporting the manipulation of numerous objects with different shapes. In the future, it could thus be deployed and tested on various physical robots.
'We demonstrated that learning a complex human-like dynamic behavior is possible using a single computer with several hours of training time,' Pavlichenko said. 'Our plans for future research involve bringing the learned model to the real world, achieving similar performance on a real robot. This is usually quite challenging, so we expect that an additional learning step, now online on the real robot, could be necessary to close the sim-to-real gap.'
© 2023 Science X Network