Clear holographic imaging in turbulent environments: Overcoming the challenges of visualizing through turbulence
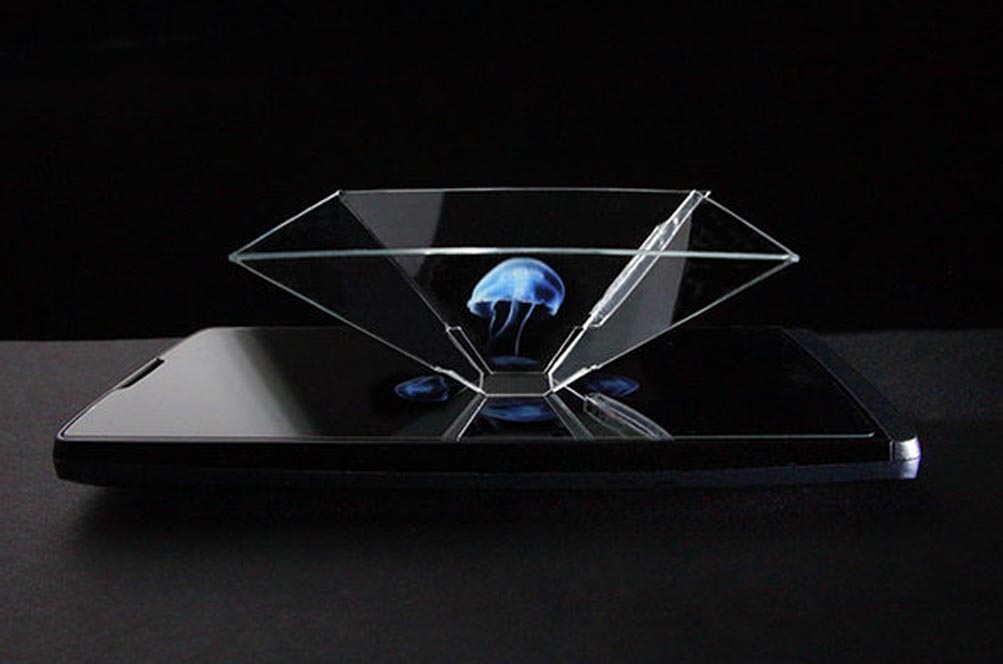
October 30, 2023
Science X's editorial team has reviewed this article, ensuring accurate and credible content by highlighting the fact-checking, peer-reviewing, referencing of trusted sources, and proofreading carried out by SPIE.
Holographic imaging has historically struggled with unpredictable distortions in various environments. The underlying issue is that traditional deep learning techniques often find adapting to broad-ranging scenes difficult due to the specific data conditions on which they rely.
Attempting to overcome this issue, researchers from Zhejiang University have investigated the intersection of optics and deep learning, revealing the importance of physical priors in lining up data and pre-trained models appropriately.
The team has examined the influence of spatial coherence and turbulence on holographic imaging, proposing a groundbreaking method named TWC-Swin for high-quality image restoration amid disturbances. The study, named 'Harnessing the magic of light: spatial coherence instructed swin transformer for universal holographic imaging,' appears in the journal Advanced Photonics.
Spatial coherence is a determinant of the orderliness of light waves. When light waves are disorderly, the resulting holographic images are blurry and noise-filled, containing less information. The preservation of spatial coherence is thus essential for high-quality holographic imaging.
Environments with a high degree of change, for instance, those with oceanic or atmospheric turbulence, generate alterations in the medium's refractive index, leading to a disturbance in the phase correlation of light waves and distortion of spatial coherence. This results in blurring, distortion, or even loss of the holographic image.
The researchers have come up with the TWC-Swin technique to handle these issues. The method, short for 'train-with-coherence swin transformer,' takes advantage of spatial coherence as a physical prior that guides the deep neural network's training. This network relies on the Swin transformer architecture, making it adept at capturing both local and global image characteristics.
To verify their method, the researchers developed a light-processing system that generated holographic images under different spatial coherence and turbulence circumstances. The holograms, based on real-world objects, served as the learning and testing data for the neural network.
The findings showed that the TWC-Swin method efficiently restores holographic images even under low spatial coherence and random turbulence conditions. This outperforms conventional convolutional network-based methods. Moreover, the method is said to have strong generalization abilities, expanding its applicability to unseen scenes not included in the training data.
This study clears the path towards tackling image degradation in holographic imaging in varied scenes. By incorporating physical principles into deep learning, the researchers highlight the successful combination of optics and computer science, paving the way for future improvements in holographic imaging, enabling us to see clearly despite turbulent conditions.
The information has been provided by SPIE and the journal concerned is Advanced Photonics.