AI-Supported Mammogram Screening Boosts Breast Cancer Detection Rate by 20%, Study Finds
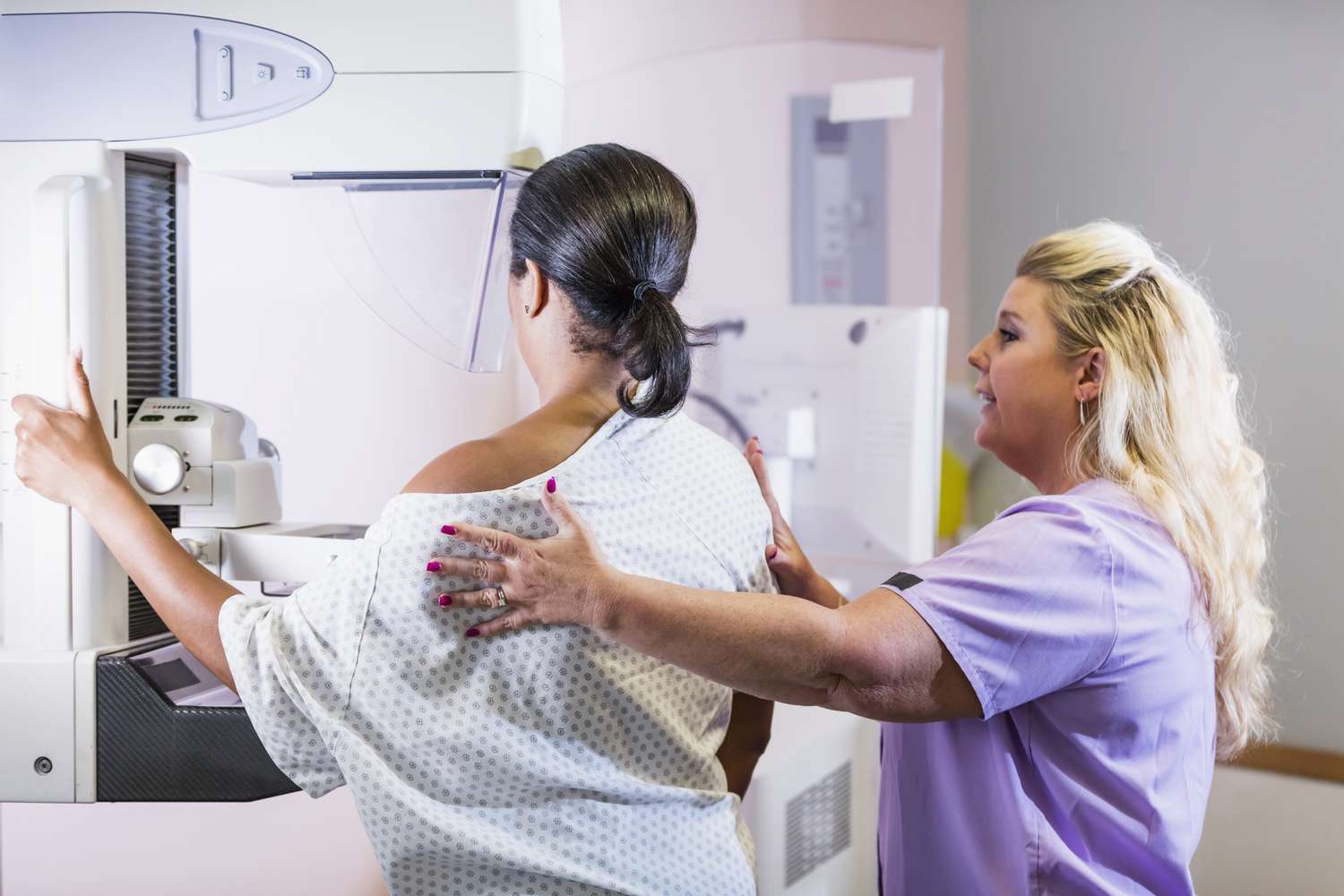
Artificial intelligence (AI) may be able to better detect breast cancer on mammogram images than trained doctors, a new study found.
The American Cancer Society recommends women who are not at higher risk for breast cancer start annual mammograms by age 45 and should have the choice to start as early as 40. There are many factors, including breast density, that can make it difficult to detect tumors with the human eye.
That said, about 1 in 8 cases of breast cancer are missed on mammograms. The new study found that AI may be able to help resolve that problem.
AI-supported breast cancer screening isn’t perfect yet, but it could help lower overburdened doctors’ workloads in the future and make mammogram readings more accurate.
“A challenging part of reading screening exams is that the vast majority of exams are normal,” said Kristina Lång, MD, PhD, an associate professor of radiology diagnostics from Lund University in Sweden, who led the study.
“When you have read hundreds of normal exams, radiologists can sometimes overlook a subtle cancer,” she said.
For the randomized, controlled, population-based trial, Lång and her team analyzed mammograms from 80,000 Swedish women who underwent the scans between April 2021 and July 2022.
Half the scans were read by two highly-trained radiologists, and the other half were read by both an experienced radiologist and AI.
The computer-assisted group detected 20% more cancers in the scans than the human-only team. In raw numbers, this works out to be a detection rate of 6 per 1,000 women screened, compared to 5 per 1,000 with standard reading.
The technology isn’t designed to be used on its own but appears to be quite useful when used in tandem with a trained eye.
Radiologists were able to detect some cases of cancer that the AI missed, and the synergistic pairing of human and computer did not increase the risk of false negatives—when a person has cancer that goes undetected during a mammogram reading.
“There is so much information contained with a mammogram image, much more than the human eye, even a highly trained human eye, can appreciate,” said Debbie Bennett, MD, Chief of Radiology, Division of Breast Imaging at Washington University in St. Louis, who was not involved in the new study.
“The number of pixels in each mammogram far exceeds what our eyes and brains can process,” she said.
AI algorithms are trained using millions of cases of breast cancer, which gives the computers a leg up when it comes to detecting abnormalities in scans, Bennett told Health.
Once an AI algorithm identifies an abnormality, it takes images of the perceived abnormality that a trained radiologist can evaluate to determine whether or not additional testing—such as an ultrasound or biopsy—is needed.
According to the University of Rochester Medical Center, about 10% of people who get a mammogram require additional testing, but only about 0.5% are found to have cancer.
In the U.S., about 240,000 cases of breast cancer are diagnosed in women every year. Early detection is paramount to outcomes. The earlier cancer is caught, the more effective treatment will likely be.
One aspect that can make it difficult to detect cancerous tumors early using mammogram images is dense breasts—something about half of women over age 40 in the U.S. have.
Dense breasts are composed of a higher amount of fibrous or glandular tissue compared to fatty tissue, which creates more white areas on a mammogram image—the same color as tumors.
That's why the Food and Drug Administration (FDA) recently amended the Mammography Quality Standards Act (MQSA) of 1992, a law passed to ensure quality mammography, to require mammography clinics to notify people who are found to have dense breasts.
Some clinics already use a computer-based calculation called volumetric assessment of breast density to determine who has dense breasts and to guide clinicians’ assessment of who needs supplemental screening. This method offers a more standardized approach to breast density, which is often left up to the discretion of each individual doctor, said Bennett.
A relatively simplified computer-based image analysis, called CAD, is also already used to pick up patterns in mammograms, which can reveal abnormalities, though it’s much less effective than AI appears to be.
Bennett noted that there’s still a lot to learn about AI.
“Because the technology is so new, there is a lack of data on the impact of AI in mammography,” she said. “We don't know what the true benefit or harm of the technology is.”
The FDA has already approved several AI technologies for breast cancer screening, but they aren’t mainstream yet. Moving forward, it’s likely that breast cancer screenings will utilize both standard methods of evaluating scans and AI.
“Currently, AI cannot replace humans,' Lång said, 'but it can make our work more effective and accurate.'
Accurate effective work is crucial for the breast cancer screening needs the United States is facing. Last year, the American College of Radiology reported both a shortage of radiologists and an aging population that requires more imaging as part of their health care.
Bennett explained that AI can really lighten the workload for an overburdened radiologist in identifying normal scans. Many scans are definitely cancer-free, and using an AI algorithm that can identify these cases with confidence would free up time for radiologists to review scans that are not definitively normal.
“That is where the expertise and clinical judgment of a trained radiologist is needed,” said Bennett.
Doctors will also still play a vital role in communicating information with patients and making judgment calls on what additional screening is appropriate for specific cases.
“Humans have to be in the steering wheel,” Lång emphasized. In Sweden, her clinic is already confident enough to begin implementing AI in breast cancer screenings.
“We know it is safe and since we performed the trial in the same setting,' she said, 'we feel certain it will work.'