Researchers Showcase Chemical Reservoir Computing via the Formose Reaction
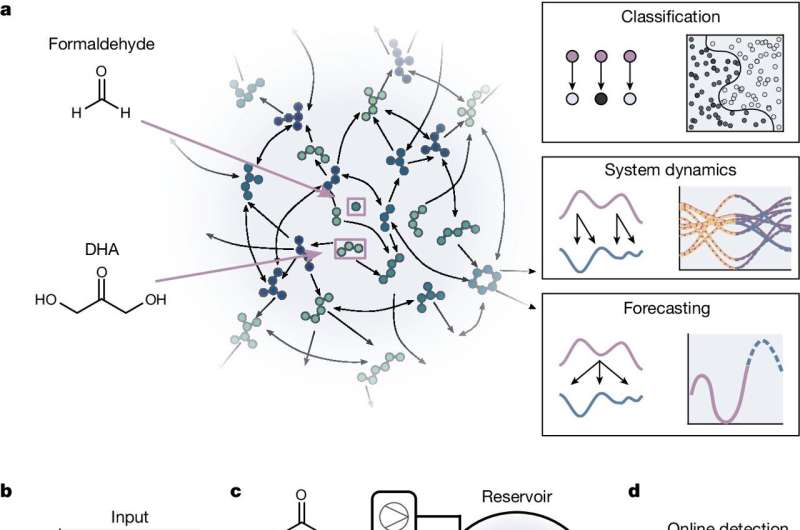
July 13, 2024 feature
This article has been reviewed according to Science X's editorial process and policies. Editors have highlighted the following attributes while ensuring the content's credibility:
- fact-checked
- peer-reviewed publication
- trusted source
- proofread
by Tejasri Gururaj , Phys.org
Researchers from the Institute for Molecules and Materials at Radboud University, Netherlands, have demonstrated that a complex self-organizing chemical reaction network can perform various computational tasks, such as nonlinear classification and complex dynamics prediction.
The field of molecular computing interests researchers who wish to harness the computational power of chemical and biological systems. In these systems, the chemical reactions or molecular processes act as the reservoir computer, transforming inputs into high-dimensional outputs.
The research, published in Nature, was led by Prof. Wilhelm Huck from Radboud University.
Researchers have tapped into the potential chemical and biological networks offer due to their complex computing abilities. However, implementing molecular computing presents challenges in terms of engineering and design.
Instead of trying to engineer molecular systems to perform specific computational tasks, Prof. Huck and his team are exploring how naturally complex chemical systems can exhibit emergent computational properties.
'I am very interested in the chemical driving forces that led to the origin of life. In this context, we are looking for mechanisms by which chemical evolution can shape the properties of complex reaction mixtures. This research drove us to consider how molecular systems can process information,' he explained to Phys.org.
The formose reaction is a chemical reaction that synthesizes sugars from formaldehyde in the presence of a catalyst, calcium hydroxide. This reaction was chosen because of its unique properties.
Prof. Huck explained, 'Although chemistry might seem complex to outsiders, most reaction sequences are rather linear. The formose reaction is the only example of a self-organizing reaction network with a highly non-linear topology, containing numerous positive and negative feedback loops.'
In other words, the reaction isn't straightforward and produces multiple intermediate compounds which react further to form new compounds. These dynamic reactions can result in a diverse set of chemical species and are non-linear in nature.
Additionally, the network includes positive feedback loops which amplify reaction outcomes, and negative feedback loops which dampen reaction outcomes.
The network is known as 'self-organizing' because it naturally evolves and reacts to the chemical inputs without the need for external intervention, producing a diverse range of outputs.
The computational capabilities emerge from the network's inherent properties rather than being explicitly programmed, making computation very flexible.
The researchers used a continuous stirred tank reactor (CSTR) to implement the formose reaction. The input concentrations of four reactants—formaldehyde, dihydroxyacetone, sodium hydroxide, and calcium chloride—are controlled to modulate the reaction network's behavior.
The output molecule is identified using a mass spectrometer, which allows them to track up to 106 molecules. This setup can be used to do calculations, with the reactant concentrations being the input value to any function that needs to be computed.
But first, the system has to be trained to find the outcome of this computation, which is done using a set of weights.
'We need to find a set of weights that converts the traces in the mass spectrometer to the correct value of the computation. This is a linear regression problem and is computationally simple. Once done, the reservoir computer computes the outcome for this function for any new input,' explained Prof. Huck.
The weights are coefficients that determine the influence of each input on the output. This training step is essential as it allows the reservoir to learn and predict how the changes in input affect the output so it can predict the output for a new set of inputs.
The researchers used the reservoir computer to do several tasks. The first was doing nonlinear classification tasks. The reservoir computer could emulate all Boolean logic gates and even tackle more complex classifications like XOR, checkers, circles, and sine functions.
The team also showed that it could predict the behavior of a complex metabolic network model of E. coli, accurately capturing both linear and nonlinear responses to fluctuating inputs across various concentration ranges.