Using reinforcement learning to make artistic collages
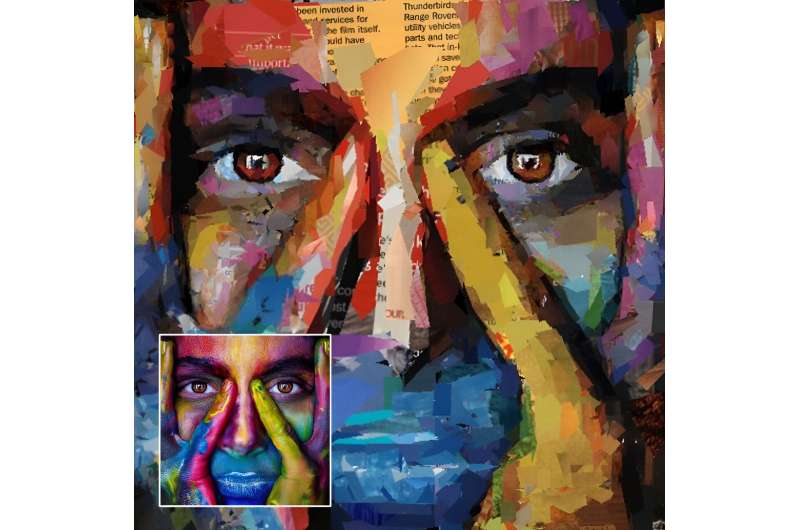
On November 26, 2023,
This feature has passed through the stringent review processes outlined by Science X's editorial policy. Furthermore, editors have confirmed that the article is:
- Fact-checked
- An arXiv preprint publication
- Sourced from a trusted outlet
- Thoroughly proofread
by Ingrid Fadelli from Tech Xplore.
A recent experiment saw a team from Seoul National University use an artificial intelligence (AI) agent to craft collages. Known as a mixed-media art form involving the assemblage of different forms to create a new whole, these collages served to imitate popular artworks and various other photographs. The team presented their innovative AI model, also detailed in a paper pre-published on arXiv, at the International Conference on Computer Vision (ICCV) 2023 in October.
The researchers explained their curiosity about the aesthetic outcome of an AI-produced collage to Tech Xplore. While current AI image generation tools can already mimic collages, they don't produce actual collages. What the team sought to create was an authentic collage, made in the traditional way.
A previous study on generating painting inspired this team to train AI using reinforcement learning (RL) to simulate human collage creation techniques. This gave them the idea of applying reinforcement learning to creating collage artworks, and hence they began building their RL-based autonomous collage artwork generator.
The goal of their research was to train an AI agent to construct collages, using methods of tearing and pasting various materials to replicate target images such as paintings and photographs, all through reinforcement learning. These collages were to be crafted using materials provided by humans.
The researchers stressed the need for the RL model to comprehend and execute the process of making a collage effectively. As reinforcement learning is essentially about learning from trial and error, the model must gain experience from working with a canvas and creating a genuine collage.
Considering the diversity of materials used in collages, a successful AI agent must test a variety of cut-and-paste options until it determines which materials result in the best likeness to the target images. Initially, the team’s model performed poorly, but over time, its capabilities showed remarkable improvement.
The researchers expressed how the reward function, defined as the increase in similarity between the collage and target image, continually evolved over time. This process enabled the AI agent to learn how to better evaluate the resemblance between its own work and the target image.
While training, the researchers instructed the model to take a random image, then try to construct a collage to mimic this image on a blank canvas. With each stage of collage-making, the agent chooses a random material and decides on cutting, scrapping, and sticking it to the canvas.
The team explained how over time, with continually changing target images and materials, the agent became adept at handling any object or image. To simplify the complex process for the existing model-free RL, the researchers developed a differentiable collaging environment that made tracking the dynamics of the collage easier, thus enhancing the model's performance.
The team's approach to training was partly inspired by previous work on RL-based painting. However, they needed to develop their own version of the model-based RL algorithm to account for the increased complexity involved in producing collages.
The authors underlined the necessity for a collage-making agent to understand the shape, texture, colors, and placement of the given material for creating the proper visual elements for the complete collage. They found that Soft Actor-Critic (SAC) was a better match for their case as it efficiently allows an agent to experience a wide array of actions in continuous action space, compared to the Deep Deterministic Policy Gradient (DDPG) used in painting.
To boost the creation of collages, the authors utilized their newly trained model as a partial collage generator unit. Interestingly, this unit was observed to produce high-resolution collage art that bore strong resemblance to a variety of target images.
'We also developed a module for analyzing target image complexity to assign more workload for partial collage generator to the place where the complexity is high,' Lee explained. 'This module can enhance the aesthetic quality of collages.'
A crucial advantage of the team's architecture is that it does not require any collage samples and demonstration data, as it was simply trained using examples of materials and target images. Notably, these materials and images are far easier to collect than original artworks.
'Without artistic data or knowledge, the agent independently learned how to make a collage,' the authors said. 'The final collaging ability was made by the agent's own exploration, which is the notable finding of this work; it shows the mighty ability of RL as a data-free learning domain.'
As the team's trained model gradually grasped the process of collage-making, it could generalize well across a wide range of images and scenarios. So far, it has only been tested in simulations. However, if applied to a humanoid robot or a robotic hand, the model could also provide 'blueprints' for the creation of physical collages.
'Building an environment in which the RL agent can learn properly was very challenging,' the authors said. 'We spent a lot of time developing and defining collage dynamics and actions that are legit for RL. Also, to save training time, we should keep them as compact and efficient as possible. Even more, we had to keep the dynamics differentiable for our model-based RL scheme as well.'
As art is highly subjective, evaluating the quality of collages produced by the model is challenging. The researchers initially carried out a user study, asking various human participants to share their opinions and feedback on the AI-created collages.
'We conducted a user study, but this may not be enough,' the authors said. 'After much consideration for more objective evaluation, we decided to use CLIP, a large vision-language pre-trained model. Because CLIP is trained with about 400M text-image pairs, we believe it has the ability to evaluate more objectively than user study. With user study and CLIP, we compared our model with other pixel-based generation models by evaluating generated images' collage-ness and content consistency.'
The user study and the CLIP-based evaluation carried out by the researchers yielded similar results. In both these tests, the new model was found to outperform other models for collage generation.
The model introduced in this recent paper could soon be developed further and tested to allow customized styles using a broader range of images and materials. In addition, the team's work could inspire the development of additional AI tools for generating various types of artwork.
'We are now interested in developing strategies that allow our models to cope with various style preferences,' the authors added said. 'As a future work, we consider developing a user-interactive interface, which can reflect user's preference during our model's creating collages.'
© 2023 Science X Network